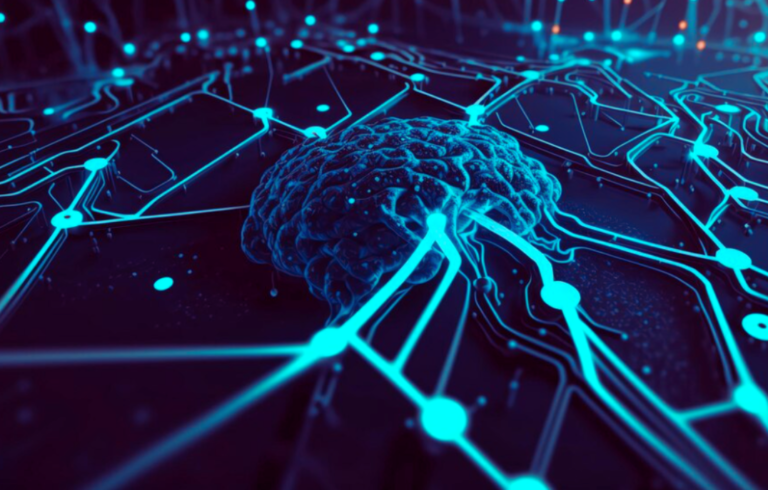
The development of new medical treatments is a long and arduous process, fraught with challenges at every step. One of the most critical stages is conducting clinical trials, where potential therapies are rigorously tested for safety and efficacy before they can be approved for widespread use. However, designing and executing these studies is a monumental undertaking, requiring careful planning, vast resources, and a deep understanding of numerous complex variables.
The costs associated with clinical trials and drug development have been rising steadily over the years. The industry is increasingly relying on artificial intelligence (AI) to support drug discovery. One of the most striking pharmaceutical industry statistics is that spending on AI will reach $3 billion by 2025 as companies invest in technology that may reduce the time and costs required to bring a new drug to market1.
Traditionally, clinical trial design has relied heavily on statistical models, expert knowledge, and past precedents. Researchers meticulously analyze data from previous studies, pore over scientific literature, and consult with subject matter experts to develop protocols that account for factors like patient demographics, disease characteristics, treatment regimens, and potential adverse events.
While this approach has led to numerous medical breakthroughs, it is not without its flaws. Human expertise, no matter how vast, is inherently limited and susceptible to bias. Statistical models, while powerful, can overlook subtle patterns or correlations hidden within the vast troves of data available. And relying too heavily on past precedents can stifle innovation and lead to the perpetuation of suboptimal practices.
Enter AI, a technology that has already transformed numerous industries and now promises to revolutionize the field of clinical research. By utilizing the capabilities of advanced algorithms, machine learning, and vast data sets, AI can augment and enhance the clinical trial design process, addressing longstanding challenges and unlocking new opportunities for innovation.
Applications of AI in Clinical Trials
1) Patient Recruitment and Selection
One of the biggest roadblocks in conducting clinical trials is identifying and enrolling an adequate number of eligible participants. Identifying and enrolling a sufficient number of suitable participants is required to ensure statistically significant results, but it is often a time-consuming and inefficient process.
Researchers must manually sift through countless medical records, applying complex inclusion and exclusion criteria to identify potential candidates.
Utilizing ML algorithms and natural language processing (NLP), AI expedites the process by sifting through vast amounts of electronic health records4, clinical notes, and patient data sources to extract crucial information, streamlining patient recruitment efforts. With remarkable speed and accuracy, AI can identify patterns and correlations that may be difficult for human researchers to discern, pinpointing individuals who meet the study criteria with precision. One study demonstrated that the system, known as the Automated Clinical Trial Eligibility Screener© (ACTES), reduced patient screening time by 34 percent and increased patient enrollment by 11.1 percent compared to manual screening of electronic health records (EHRs). The system also improved the number of patients screened by 14.7 percent and those approached by 11.1 percent5.
Furthermore, AI can help enlist under-represented populations based on factors such as age, gender, and medical history to ensure a diverse study sample. This enhances result generalizability and enables nuanced analyses considering factors such as disease subtypes, comorbidities, and demographic variations.
2) Study Design and Protocol Development
Study design and protocol development can greatly benefit from the use of AI, particularly in the field of optimal randomization, which helps to eliminate bias and ensures that treatments are distributed evenly. It goes far beyond just finding patients to enroll. It extends well beyond patient recruitment as well. AI can find potential confounding factors, ideal treatment plans, and potential adverse events that human researchers might have missed, by ingesting and analyzing data from a variety of sources, including previous clinical trials, scientific literature, real-world evidence, and even social media and patient forums.
AI can even assist in selecting specific goals and outcome measures, ensuring that the study captures highly relevant and clinically significant data for thorough analysis.
3) Adaptive Trial Design
The dynamic nature of clinical research demands flexibility. Unlike traditional fixed designs, adaptive trials allow for real-time modifications to certain aspects of the study based on interim results or emerging data. This flexibility can lead to more efficient and informative studies. Adaptive trials, are a recently proposed approach to shorten the phases of clinical trials, decrease the required number of patients for enrollment, enhance predictions of later drug success, and lower drug development costs6.
AI can help researchers navigate this complexity by simulating various scenarios, such as changes in patient demographics or unexpected treatment outcomes, and providing data-driven recommendations for adaptations. For instance, AI algorithms can analyze interim data and suggest adjustments to sample sizes, treatment arms, or endpoint selection based on predetermined decision rules. This real-time optimization can ensure that the study remains on track to achieve its objectives while minimizing the risk of wasting resources or exposing participants to unnecessary risks.
4) Data Quality Assurance
The accuracy and reliability of data are fundamental to dependable clinical research and development initiatives. Inaccurate or incomplete data can lead to flawed results, potentially putting patients at risk and wasting valuable time and resources. A study reported that overall 20% of trials inconsistently reported the primary outcome result, although it noted that only a few discrepancies could be considered potentially meaningful6. Traditionally, data quality assurance has relied heavily on manual processes, such as source data verification and statistical monitoring, which can be time-consuming and prone to human error.
Through automation, AI enhances data quality by automating data validation checks, error detection, and data cleaning processes, ensuring the accuracy and reliability of collected data for thorough analysis. For example, AI systems can be trained to identify anomalies, inconsistencies, or missing data in real-time, flagging potential issues for human review and intervention. Additionally, AI can assist in data cleaning and standardization, ensuring that the data is consistent and ready for analysis, minimizing the need for extensive manual data processing.
5) Continuous Monitoring
Continuous monitoring of clinical trials is another area where AI can be very useful. By analyzing data as it is collected, AI algorithms can identify potential safety concerns, adverse events, or deviations from the study protocol. Enabling early detection of potential concerns, real-time monitoring through AI empowers researchers to swiftly implement proactive measures, ensuring participant safety through timely interventions.
6) Cost Reduction and Accelerated Development
While AI offers numerous benefits in clinical trial design and execution, its true value lies in its potential to reduce costs and accelerate the development of new therapies. In the clinical research phase, AI can cut costs by $28 billion per year, and reduce the phase’s length by half or more. AI does this by automating manual tasks and performing rapid statistical analysis to improve clinical research efficiency. The increasing use of AI in the pharmaceutical industry will not only reduce costs, but enable more effective drugs to be created more quickly, boosting medical innovations and leading to a healthier world7. By optimizing patient recruitment, enhancing study design, facilitating adaptive trials, improving data quality, and enabling continuous monitoring, AI can help streamline processes and reduce the time and resources required to conduct these critical studies.
More efficient patient recruitment can significantly reduce the costs associated with screening and enrolling participants, which can account for a substantial portion of a clinical trial’s budget. Optimized study design can minimize the risk of costly protocol amendments or study delays, while improved data quality can reduce the need for extensive data cleaning and decrease the likelihood of needing to repeat portions of the study due to data issues.
Additionally, researchers may be able to shorten the length of clinical trials by using AI for real-time monitoring to quickly spot adverse events and adaptive trial design to make quick changes. This could mean that new therapies reach the market more quickly. This not only translates to cost savings for pharmaceutical companies and research organizations but also means that potentially life-saving treatments can reach patients more quickly.
Collaboration between AI and Human Expertise
It is important to recognize that AI is a powerful tool to augment human expertise, but it is not a replacement for human judgment and ethical considerations in clinical research. Rather, AI serves as a powerful tool that complements and enhances the capabilities of human researchers in clinical trial design. AI systems, no matter how advanced, are ultimately tools designed to augment and enhance the capabilities of human researchers and clinicians.
While AI can provide valuable insights and recommendations, it will always be up to human experts to interpret and contextualize these outputs, weighing them against their own knowledge, experience, and ethical considerations. Clinical trials, after all, are not merely scientific exercises but also involve complex ethical and moral dimensions, as they directly impact the lives and well-being of human participants.
As such, the integration of AI in clinical trial design and execution should be viewed as a collaborative effort where human researchers and AI systems work in tandem, each leveraging their respective strengths to drive innovation and advance medical knowledge.
Ethical Considerations and Governance
Robust governance frameworks, ethical standards, and regulatory oversight are necessary for the implementation of AI in clinical research, as with any transformative technology. As with any powerful technology, there are valid concerns around issues such as data privacy, algorithmic bias, and the potential for AI systems to perpetuate or amplify existing societal biases and inequalities.
Transparency, accountability, and ethical responsibility must be the guiding principles for the development and use of AI in clinical research. Researchers and organizations must ensure that AI systems are developed and used in a manner that prioritizes patient safety, upholds ethical standards, and promotes equitable access to healthcare.
Notwithstanding these obstacles, we cannot overlook the substantial advantages that AI could bring to the design and execution of clinical trials. With healthcare spending on the rise, demand for services on the rise, and the constant need to innovate, artificial intelligence (AI) offers a formidable answer to persistent problems.
By using advanced algorithms, ML, and extensive data, researchers can surpass the constraints of conventional methods, exploring new areas in medical research and speeding up the creation of life-saving therapies.
Incorporating AI into clinical research signifies more than just technological progress; it marks a fundamental change that can revolutionize how we engage in medical innovation, ultimately improving patient outcomes worldwide.
Researchers, clinicians, policymakers, and society at large must accept AI with responsibility and ethics as we enter an age where it will play an ever-increasing role in healthcare. We must ensure that this technology reaches its maximum potential while preserving the fundamental principles of medicine, which include beneficence, non-maleficence, autonomy, and justice.
References